User guide
Level 0 to Level 3 processing
Two components are needed to perform Level 0 to Level 3 processing: - A Level 0 dataset file (.txt), or a collection of Level 0 dataset files - A station config file (.toml)
Two test station datasets and config files are available with pypromice as an example of the Level 0 to Level 3 processing. These can be found on the Github repo here, in the src/pypromice/test/
directory in the cloned repo.
These can be processed from Level 0 to a Level 3 data product as an AWS
object in pypromice.
from pypromice.process import AWS
# Define input paths
config = "src/pypromice/test/test_config1.toml"
inpath = "src/pypromice/test/"
# Initiate and process
a = AWS(config, inpath)
a.process()
# Get Level 3
l3 = a.L3
All processing steps are executed in AWS.process
. These can also be broken down into each Level processing
from pypromice.process import AWS
# Define input paths
config = "src/pypromice/test/test_config2.toml"
inpath = "src/pypromice/test/"
# Initiate
a = AWS(config, inpath)
# Process to Level 1
a.getL1()
l1 = a.L1
# Process to Level 2
a.getL2()
l2 = a.L2
# Process to Level 3
a.getL3()
l3 = a.L3
Level 3 data can be saved as both NetCDF and csv formatted files using the AWS.write
function.
a.write("src/pypromice/test/")
The Level 0 to Level 3 processing can also be executed from a CLI using the getL3
command.
$ get_l3 -c src/pypromice/test/test_config1.toml -i src/pypromice/test -o src/pypromice/test
Loading our data
Import from Dataverse (no downloads!)
The automated weather station (AWS) datasets from the PROMICE and GC-Net monitoring programmes are openly available on the GEUS Dataverse. These can be imported directly with pypromice, with no downloading required.
import pypromice.get as pget
# Import AWS data from station KPC_U
df = pget.aws_data("kpc_u_hour.csv")
All available AWS datasets are retrieved by station name. Use aws_names()
to list all station names which can be used as an input to aws_data()
.
n = pget.aws_names()
print(n)
Download with pypromice
AWS data can be downloaded to file with pypromice. Open up a CLI and use the getData
command.
$ get_promice_data -n KPC_U_hour.csv
Files are downloaded to the current directory as a CSV formatted file. Use the -h
help flag to explore further input variables.
$ get_promice_data -h
Note
Currently, this functionality within pypromice is only for our hourly AWS data. For daily and monthly AWS data, please download these from the GEUS Dataverse.
Load from NetCDF file
AWS data can be loaded from a local NetCDF file with xarray
.
import xarray as xr
ds = xr.open_dataset("KPC_U_hour.nc")
Load from CSV file
AWS data can be loaded from a local CSV file and handled as a pandas.DataFrame
.
import pandas as pd
df = pd.read_csv("KPC_U_hour.csv", index_col=0, parse_dates=True)
If you would rather handle the AWS data as an xarray.Dataset
object then the pandas.DataFrame
can be converted.
ds = xr.Dataset.from_dataframe(df)
Plotting our data
Once loaded, variables from an AWS dataset can be simply plotted with using pandas or xarray.
# Plot variable with pandas
# In this case, we will plot air pressure
df.plot(kind='line', y='p_u', use_index=True)
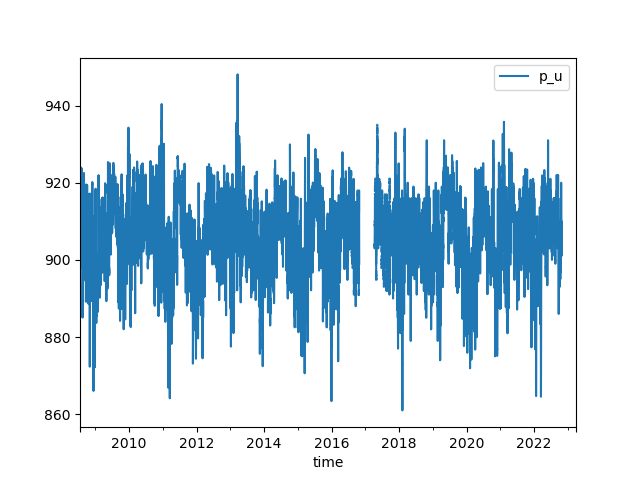
# Plot variable with xarray
# In this case, we will plot air temperature
ds['t_u'].plot()
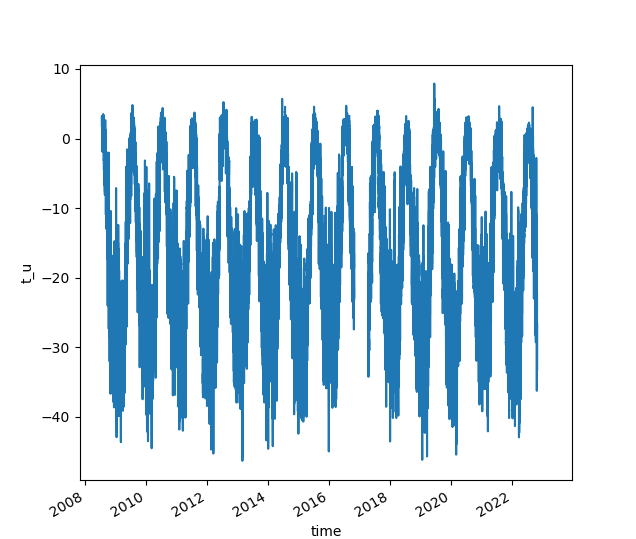
Note
Variable names are provided in the dataset metadata, or can be found on in our variables look-up table. For more complex plotting, please see either the xarray or pandas plotting documentation.
Warning
Plotting with either xarray or pandas requires matplotlib. This is not supplied as a dependency with pypromice, so please install matplotlib separately if you wish to do so.